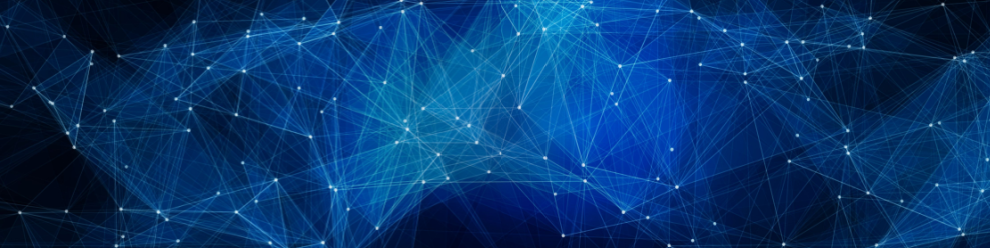
Generative Artificial Intelligence (Gen AI) is a disruptive technology, taking over industries worldwide. While AI has been around for some time now, Gen AI is a newer type of AI that uses deep learning and neural networks to create its own content. With its ability to innovate, automate, and personalize outputs, it has the potential to immensely benefit the financial services industry.
We spoke with Paul Fahey, Head of Investment Data Science at Northern Trust, and Sandeep Varma, Co-Founder and CEO at Equity Data Science (EDS), about the evolution of AI in financial services and how they see it impacting the industry in the future.
How has AI evolved in the financial services industry?
Paul Fahey: Artificial intelligence has been around for many years, and its evolution has been nothing short of transformative. Developed back in the 1950’s, it was a rudimentary technology compared to what it is today. It started to pick up traction in the financial services industry in the 1980’s and ‘90’s with some of its first use cases including reducing the risk of human mistakes, fraud detection, predictive analysis and chatbots. Now, AI has grown from the simpler forms of machine learning to Gen AI and large language models, which have the capacity to learn patterns and structures to create new content. AI is revolutionizing how businesses operate and how data is analyzed. It has paved the way for increased accuracy, efficiency and accessibility, setting the stage for the vast potential that Gen AI has in financial services.
Sandeep Varma: AI has significantly evolved in the financial services industry over the years, changing the way businesses operate and serve their clients. I think most people think of AI as an evolution in automation which has been going on for many decades, however it is much more than that. While AI and automation both allow organizations to operate more efficiently and effectively, automation is simply allowing a process to operate by itself, without human intervention. AI, on the other hand, is the science of developing intelligent machines that have the ability to learn patterns in datasets which can assist in automation.1 In the financial services industry, we have seen a number of AI use cases such as the automation of applications (think of mortgage or loan applications), detecting fraudulent credit card activity and improving customer service. Generative AI is a leap over these traditional AI use cases and will play an even more prominent role in shaping the future of financial services.
What has been the evolution of AI at Northern Trust?
Fahey: Northern Trust has been developing and leveraging AI for more than 10 years with rapid acceleration in the last five years. Initially, it was being used for automation, deploying Robotic Process Automation (RPA) to reduce or eliminate manual tasks. Since then, we have harnessed the technology to expand into machine learning (ML) and execute basic “decision” activities, further automating operational activities. With the development of Gen AI, Northern Trust is now looking into potential use cases for the technology in order to deliver value to the front office of our clients.
What inspired Equity Data Science (EDS) to incorporate generative AI into its business model?
Varma: We were in the early stages of redeveloping our Research Management System (RMS) when ChatGPT took the world by storm. A research management system houses all of the qualitative research produced by an investment firm and Gen AI seemed like the perfect tool to add to our product offering to help investors gain insight from their most valuable research data. It was clear to us that RMSs not incorporating Gen AI in their offering would be at a competitive disadvantage.
How do you foresee generative AI impacting investment data analysis in the future, and what specific benefits or opportunities do you expect it to bring to the investment industry?
Fahey: Gen AI has the potential to revolutionize investment data analysis. It has the capability to create advanced predictive models to determine plausible market outcomes, assess risks and opportunities and provide valuable insights. As a result, subject matter experts’ (SME) capacity will likely not be limited by the number of hours in a day. Much like the printing press enabled mass production of books and increased accessibility, Gen AI has the potential to significantly increase the capacity of SMEs.
Varma: It's easy to see the productivity benefits of Generative AI, including summarizing investor and industry call transcripts and being able to ask questions of the plethora of data without having to comb through all of it. Insight and action-oriented use cases will emerge as the quant funds deploy Gen AI to predict markets and trade on it with greater confidence. This will enable investors to stress-test their portfolios and develop more robust investment strategies. Overall, Gen AI presents many opportunities for investment data analysis, which will significantly improve current processes and reduce the time it takes to complete tasks.
As generative AI models become more sophisticated, what challenges do you anticipate? For example, is there a potential for bias in the investment decision-making processes?
Fahey: While the potential of Gen AI in investment data science is vast, it does not come without its challenges. As more companies adapt Gen AI, there will be increased regulations around the technology which may pose a challenge. External input and outputs will also need to be properly managed. External inputs risk “hallucinations”, which are incorrect or false outputs. External outputs also risk exposing internal data, making data privacy a challenge. Humans in the loop (HITL) will play a key role in mitigating the risks inherent in Gen AI. Two key roles in the successful deployment of large language models (LLMs); writing effective prompts and interpreting the outputs, remain the domain of the human in the loop.
Varma: Gen AI may also present challenges related to data quality and ethics. Generating high-quality synthetic data that accurately reflects real-world scenarios can be difficult, as Gen AI may unintentionally introduce biases or inaccuracies. Additionally, ethical concerns arise when using Gen AI, such as ensuring the responsible handling of synthetic data, addressing privacy implications and guarding against potential misuse.
How is EDS using generative AI today? What are your future plans?
Varma: Within EDS, we are using Gen AI to:
- Summarize and store calls, transcripts and content
- Auto-tag research notes to the appropriate securities and themes
- Answer questions about notes and analyze those notes
For our Research Management capability, we are planning to use it to:
- Auto tag with smart logic and sentiment tagging - “I want you to tag each note with one of these five tags. You determine which one goes to which note.”
- Automate ticker tagging to more thoroughly organize data
- Fuzzy search - type in a query and it'll bring up notes that are relevant to that query even if the specific words aren't in there
- Ask questions in Slack chat for internal use cases such as new employee and helpdesk training
Another idea for the future is to leverage Gen AI for marketing materials, such as an automated newsletter that could be very fund specific. Gen AI allows you to do somewhat complex activities at scale (GPT-type LLMs).
As generative AI continues to evolve, how do you plan to integrate the latest advancements and ensure that your clients benefit from cutting-edge capabilities?
Varma: EDS is already integrating the latest advancements with the use of GPT-4. GPT-4 represents a significant advancement in Gen AI, offering improved natural language understanding, context retention and the ability to generate even more coherent and contextually relevant text.
We are also looking at Gen AI for its potential data analysis capabilities. For example, how can it identify portfolio risk in client portfolios? Or how can it more efficiently analyze a client’s Excel workbooks and pull out the most important data? It isn't limited to chatbot functionality. Think of Gen AI as a brain to enhance processes and products.
Our main goal as a business is to present our clients’ vision with clarity and precision, which requires transparency and good user experiences. Everything we do is about providing clients access to their information and providing the analysis clients need to help them make decisions better and Gen AI is another interesting tool to bring them to that goal.
As more cutting-edge capabilities become available, EDS will assess the benefits and risks to determine whether integrating them into our product offering is the right choice.
Fahey: While Northern Trust is not currently integrating Gen AI into client facing applications, the financial services industry has seen a remarkable evolution in AI technologies. It has revolutionized how businesses operate to meet their clients’ needs, and Northern Trust continues to research and invest in this technology to make our processes more efficient. The desire to incorporate Gen AI into business models will continue to grow due to its capacity to manage data, streamline content creation, enhance risk assessment and foster innovation. Successful execution will be heavily dependent on the development of the human skillsets and the quality and the quantity of the data underpinning the models.
1 What is Artificial Intelligence (AI) ? | IBM